Personalized Marketing at Scale: How AI Predictive Models Are Transforming Large-Scale Campaigns
- AI Content Strategist
- Oct 29, 2024
- 3 min read
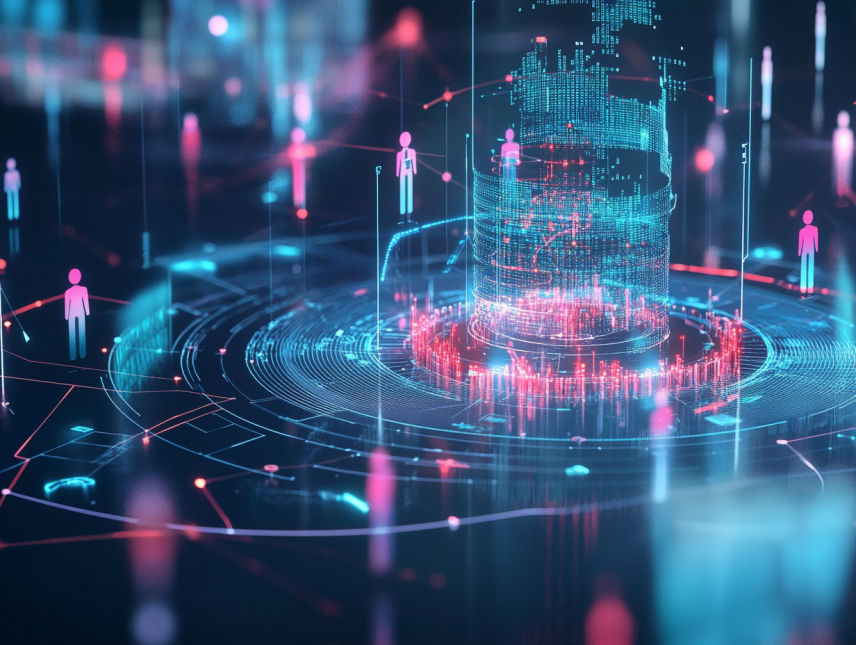
In an era where consumer expectations are constantly evolving, brands are increasingly challenged to deliver personalized marketing experiences at scale. The rise of AI predictive models has revolutionised the way companies approach large-scale marketing campaigns, enabling them to tailor content and strategies to individual preferences while managing extensive customer bases. This article explores how AI predictive models are transforming personalized marketing, allowing businesses to automate hyper-personalized strategies and enhance their customer relationship management (CRM) capabilities.
The Challenge of Personalization at Scale
Personalization is no longer a luxury; it’s a necessity. Today's consumers demand experiences that resonate with their individual preferences and behaviours. However, achieving this level of personalization across large customer bases presents significant challenges. Marketers often struggle to balance relevance with scalability, leading to generic campaigns that fail to engage audiences. This is where AI predictive models come into play.
The Role of AI Predictive Models in Marketing
AI predictive models leverage advanced algorithms and machine learning techniques to analyse vast amounts of customer data. Here’s how they contribute to scaling personalized marketing campaigns:
Data Analysis and Pattern Recognition: AI tools can process and analyse diverse datasets—from purchase histories and browsing behaviours to demographic information. By identifying patterns and trends within this data, predictive models enable marketers to segment audiences more effectively and understand their unique preferences.
Hyper-Personalized Marketing Strategies: With insights derived from data analysis, brands can automate hyper-personalized marketing strategies. For instance, AI can predict which products a customer is likely to purchase based on their past interactions, allowing for tailored recommendations that significantly enhance the customer experience.
Dynamic Content Delivery: AI predictive models can facilitate dynamic content delivery, ensuring that the right message reaches the right audience at the right time. This means that brands can create targeted email campaigns, personalised web experiences, and relevant social media ads, driving higher engagement and conversion rates.
Integrating AI with CRM Platforms
To enhance scalability and personalization in large-scale marketing efforts, the integration of AI with CRM platforms is crucial. Here’s how this integration benefits businesses:
Centralized Data Management: Integrating AI predictive models with CRM systems enables businesses to centralise customer data, providing a comprehensive view of each customer’s journey. This facilitates more effective segmentation and targeting.
Automated Campaign Management: AI-driven automation tools can streamline campaign management processes, allowing marketers to launch, monitor, and adjust campaigns with minimal manual intervention. This increases efficiency and reduces the risk of human error.
Real-Time Insights and Adaptability: The combination of AI and CRM platforms offers real-time insights into customer behaviour, enabling marketers to adapt their strategies quickly. For example, if a sudden trend emerges, brands can pivot their messaging to capitalise on the opportunity.
Case Studies: AI in Action
Several brands have successfully harnessed AI predictive models to transform their marketing campaigns:
Netflix: As a pioneer in personalised content recommendations, Netflix utilises AI algorithms to analyse viewer preferences and viewing patterns. This allows the platform to offer tailored content suggestions, resulting in increased viewer engagement and retention.
Amazon: Amazon’s recommendation engine is a prime example of AI-driven personalization at scale. By analysing vast amounts of customer data, Amazon delivers hyper-personalized product recommendations, contributing significantly to its sales and customer loyalty.
Coca-Cola: Coca-Cola leverages AI to analyse customer interactions and preferences, allowing the company to create targeted marketing campaigns. Through AI-driven insights, Coca-Cola can deliver relevant content to its diverse customer base, enhancing engagement and brand loyalty.
Best Practices for Implementing AI Predictive Models
To maximise the benefits of AI predictive models in personalized marketing, businesses should consider the following best practices:
Invest in Quality Data: The effectiveness of AI predictive models depends on the quality of data. Brands must ensure they collect accurate, comprehensive, and up-to-date customer information.
Prioritise Customer Privacy: As personalization relies heavily on customer data, brands must prioritise data privacy and transparency. Ensuring compliance with data protection regulations will help build trust with customers.
Continuously Optimise Models: AI predictive models should be continually refined and optimised based on performance metrics and changing consumer behaviours. Regular assessments will ensure that marketing strategies remain relevant and effective.
Leverage Cross-Channel Strategies: Brands should adopt cross-channel marketing strategies that integrate AI-driven personalization across various platforms, ensuring a cohesive and seamless customer experience.
Conclusion
As consumer expectations for personalised experiences continue to rise, AI predictive models are proving to be invaluable tools for marketers seeking to scale their efforts. By analysing vast amounts of customer data and automating hyper-personalized strategies, brands can effectively engage their audiences while maintaining relevance.
The integration of AI with CRM platforms further enhances the scalability and effectiveness of personalized marketing campaigns, allowing businesses to adapt quickly to changing market dynamics. As AI technology advances, the future of personalized marketing will be shaped by these innovative approaches, enabling brands to create meaningful connections with their customers on a large scale.
Comments